Keynote Speakers
Prof. Nikola Kasabov
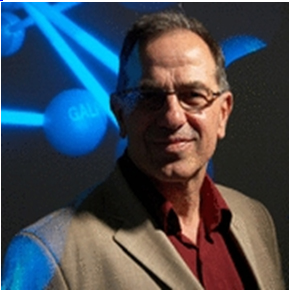
- Director and Founder, Knowledge Engineering and Discovery Research Institute (KEDRI)
- Chair of Knowledge Engineering, Auckland University of Technology
- Institute for Neuroinformatics - ETH
- University of Zurich
Subject: “Neurocomputing for Spatio/Spectro-Temporal Pattern Recognition and Early Event Prediction: Methods, Systems, Applications”
Abstract
The talk presents a brief overview of contemporary methods for neurocomputation, including: evolving connections systems (ECOS) and evolving neuro-fuzzy systems [1]; evolving spiking neural networks (eSNN) [2-5]; evolutionary and neurogenetic systems [6]; quantum inspired evolutionary computation [7,8]; rule extraction from eSNN [9]. These methods are suitable for incremental adaptive, on-line learning from spatio-temporal data and for data mining. But the main focus of the talk is how they can learn to predict early the outcome of an input spatio-temporal pattern, before the whole pattern is entered in a system. This is demonstrated on several applications in bioinformatics, such as stroke occurrence prediction, and brain data modeling for brain-computer interfaces [10], on ecological and environmental modeling [11]. eSNN have proved superior for spatio-and spectro-temporal data analysis, modeling, pattern recognition and early event prediction as outcome of recognized patterns when partially presented.
Future directions are discussed. Materials related to the lecture, such as papers, data and software systems can be found from http://www.kedri.aut.ac.nz and also from http://www.theneucom.com and http://ncs.ethz.ch/projects/evospike/.
References
- N.Kasabov (2007) Evolving Connectionist Systems: The Knowledge Engineering Approach, Springer, London (www.springer.de) (first edition published in 2002)
- S.Wysoski, L.Benuskova, N.Kasabov, Evolving Spiking Neural Networks for Audio-Visual Information Processing, Neural Networks, vol 23, issue 7, pp 819-835, September 2010.
- N.Kasabov, To spike or not to spike: A probabilistic spiking neural model, Neural Networks, 23, 1, 2010, 16-19.
- Mohemmed,A., Schliebs,S., Kasabov,N.(2011),SPAN: Spike Pattern Association Neuron for Learning Spatio-Temporal Sequences, Int. J. Neural Systems, 2012.
- Kasabov, N., Dhoble, K., Nuntalid, N., G. Indiveri, Dynamic Evolving Spiking Neural Networks for On-line Spatio- and Spectro-Temporal Pattern Recognition, Neural Networks, v.41, 2013, 188-201.
- Benuskova, L and N.Kasabov (2007) Computaional Neurogenetic Modelling, Springer.
- Defoin-Platel, M., S.Schliebs, N.Kasabov, Quantum-inspired Evolutionary Algorithm: A multi-model EDA, IEEE Trans. Evolutionary Computation, vol.13, No.6, Dec.2009, 1218-1232
- Nuzly, H., N.Kasabov, S.Shamsuddin (2010) Probabilistic Evolving Spiking Neural Network Optimization Using Dynamic Quantum Inspired Particle Swarm Optimization, Proc. ICONIP 2010, Part I, LNCS, vol.6443.
- S.Soltic, N.Kasabov, Knowledge extraction from evolving spiking neural networks with a rank order population coding, Int.J.Neural Systems, Vol. 20, No. 6 (2010) 437-445, World Scientific Publ.
- N.Kasabov (ed) The Springer Handbook of Bio- and Neuroinformatics, Springer, 2013.
- Schliebs, Michael Defoin Platel, Susan Worner and Nikola Kasabov, Integrated Feature and Parameter Optimization for Evolving Spiking Neural Networks: Exploring Heterogeneous Probabilistic Models, Neural Networks, 22, 623-632, 2009.
Prof. Marios M. Polycarpou
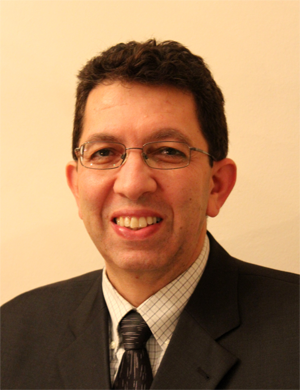
- IEEE Fellow
- President, IEEE Computational Intelligence Society
- Director, KIOS Research Center for Intelligent Systems and Networks
- Dept. of Electrical and Computer Engineering
- University of Cyprus
Subject: “Distributed Sensor Fault Diagnosis in Big Data Environments”
Abstract
The emergence of networked embedded systems and sensor/actuator networks has given rise to advanced monitoring and control applications, where a large amount of sensor data is collected and processed in real-time in order to achieve smooth and efficient operation of the underlying system. The current trend is towards larger and larger sensor data sets, leading to so called big data environments. However, in situations where faults arise in one or more of the sensing devices, this may lead to a serious degradation in performance or even to an overall system failure. The goal of this presentation is to motivate the need for fault diagnosis in complex distributed dynamical systems and to provide a methodology for detecting and isolating multiple sensor faults in a class of nonlinear dynamical systems. The detection of faults in sensor groups is conducted using robust analytical redundancy relations, formulated by structured residuals and adaptive thresholds. Various estimation algorithms will be presented and illustrated, and directions for future research will be discussed.
Prof. Erkki Oja
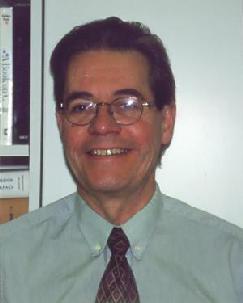
- Recipient of the 2006 IEEE Computational Intelligence Society Neural Networks Pioneer Award.
- Director of the Adaptive Informatics Research Centre,
- Chairman of the Finnish Research Council for Natural Sciences and Engineering,
- Visiting Professor at the Tokyo Institute of Technology, Japan
- Member of the Finnish Academy of Sciences,
- IEEE Fellow,
- Founding Fellow of the International Association of Pattern Recognition (IAPR),
- Past President of the European Neural Network Society (ENNS),
- Fellow of the International Neural Network Society (INNS)
- Author of the scientific books:
- "Subspace Methods of Pattern Recognition" New York: Research Studies Press and Wiley, 1983, translated into Chinese and Japanese;
- "Kohonen Maps" Amsterdam: Elsevier, 1999
- "Independent Component Analysis" New York: Wiley, 2001 translated in Chinese and Japanese.
- His research interests include principal component and independent component analysis, self-organization, statistical pattern recognition, and applying artificial neural networks to computer vision and signal processing.
- Aalto University, Finland
Subject: “Machine learning for big data analytics”
Abstract
During the past 30 years, the amount of stored digital data has roughly doubled every 40 months. Today, about 2.5 quintillion bytes are created very day. This data comes from sensor networks, cameras, microphones, mobile devices, software logs etc. Part of it is scientific data especially in particle physics, astronomy and genomics, part of it comes from other sectors of society such as internet text and documents, web logs, medical records, military surveillance, photo and video archives and e-commerce. This data poses a unique challenge in data mining: finding meaningful things out of the data masses. Central algorithmic techniques to process and mine the data are classification, clustering, neural networks, pattern recognition, regression, visualization etc. Many of these fall under the term machine learning. In the author's research group at Aalto University, Finland, machine learning techniques are developed and applied to many of the above problems together with other research institutes and industry. The talk will cover some recent algorithmic discoveries and illustrate the problem area with case studies in speech recognition and synthesis, video recognition, brain imaging, and large-scale climate research.
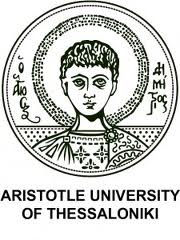
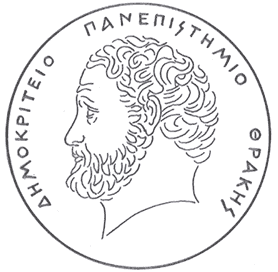
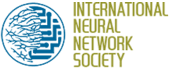
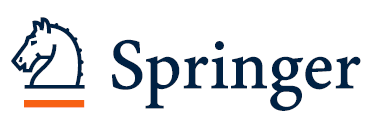
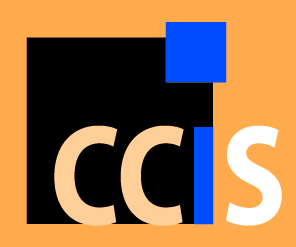